Computational Vision & Machine Intelligence
Computational Imaging Lab
Deep Learning Reading Group (DLRG)
Funded projects
My research focuses on advancing artificial intelligence in medical imaging, particularly in understanding how tumors influence tissue texture. Medical imaging modalities capture diverse texture patterns, and a single approach often falls short in analyzing such heterogeneity. By integrating deep learning techniques with texture analysis and fractal characterization, my work aims to identify subtle abnormalities and distinguish tumor subtypes early. These methods not only enhance the detection and classification of tumors but also enable precise assessment of their progression or regression, paving the way for more effective and informed decision-making in clinical practice.
The first texture is represented by images acquired noninvasively giving a fine texture structure, where lung tumor contrast enhanced images were acquired via computed tomography (CT) modality. Lung tumor staging predication accuracy was improved from conventional CT alone i.e. without using a PET scan, through identifying malignant aggressiveness of lung tumors by examining vascularized tumor regions that exhibit strong fractal characteristics. (more info)
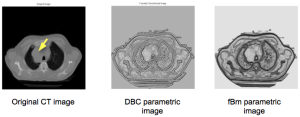
The quality of the extracted texture feature is substantial for an accurate diagnosis. Therefore the impact of noise extracted via different statistical, model and filtered-based texture analysis algorithms was assessed on non-contrast enhanced CT images. Experiments were performed on lung tumor regions of interest, and the performance of the texture analysis method was compared without and under noise presence. (more info)
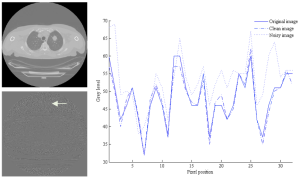
In another work, a different type of texture was dealt with for the purpose of developing an automated system for improving histopathological meningioma brain tumors discrimination acquired invasively via digital microscopy modality. Histopathological texture usually has a macro or coarse structure where the cell nuclei color, shape and orientation defines the general texture structure and play an important role in the feature extraction process (more info) and for automated classification (more info, video).
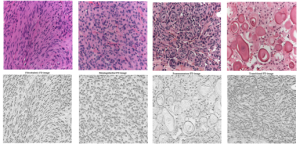
My work was also concerned with a third type of texture represented in the problem of ultrasound tissue characterization. Tumor spatial and contrast resolution in ultrasound images is low as compared to other modalities, therefore a new approach for assessing subtle heterogeneities within a given mass in ultrasound image texture was proposed. Volumetric Nakagami-based shape and scale parameters were estimated from ultrasound RF, and a multi-resolution Daubechies wavelet packet transform was performed adaptively. Finally, local multi-scale textural fractal descriptors were extracted from volumetric patches. Results show improved prediction of therapy response and tumor characterization. (more info)
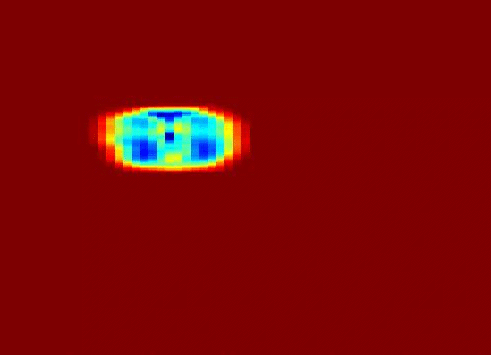
Also fractal analysis of the echo signal was found to give additional information about the heterogeneity of the underlying liver tissue structure. Regions within the liver tumor tissue which responded to chemotherapy treatment were shown to exhibit different statistical properties to that of the non-respondent counterpart. (more info)
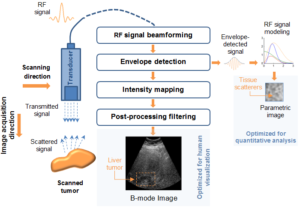
In the realm of myocardial segmentation, a novel algorithm was proposed for correct left ventricle (LV) segmentation in 3D echocardiographic images sequences based on fractional Brownian motion. Dealing with the LV segmentation problem from a spatio-temporal perspective can give further information on the shape boundaries. (more info)
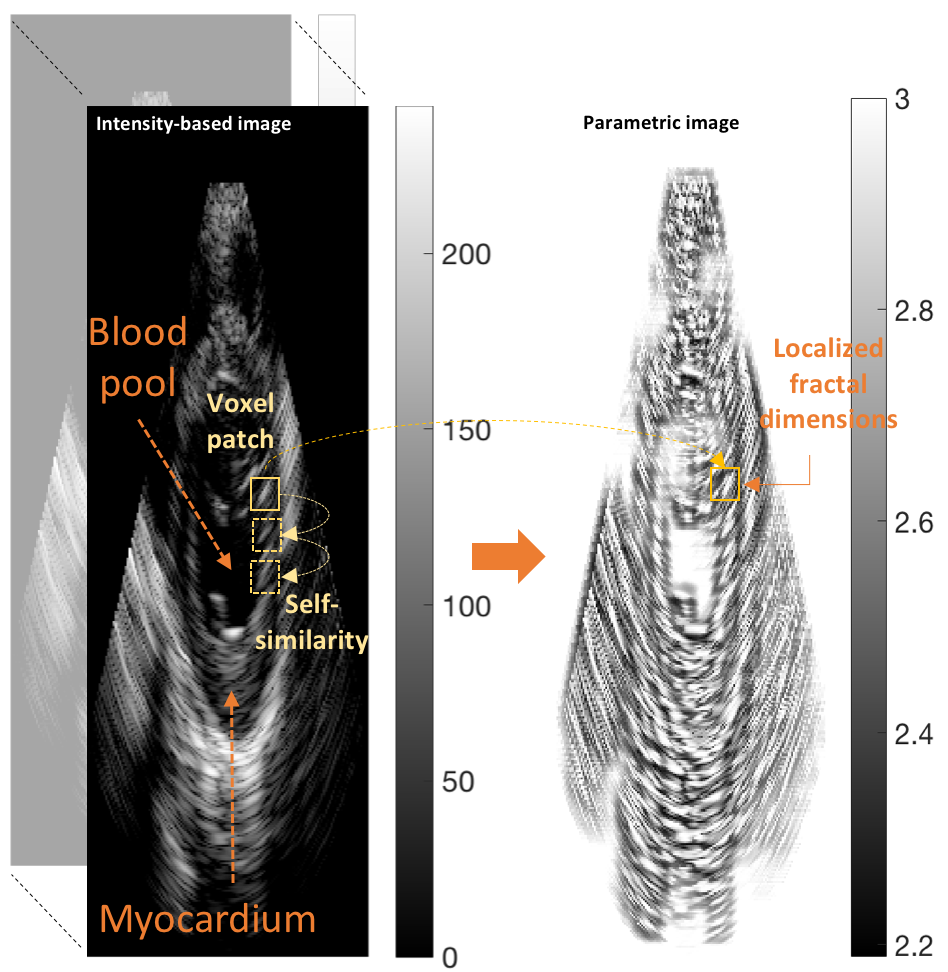